Summary
What are the possibilities of Computational methods, such as NLP and Deep Learning AI, for the study of history? This question is at the heart of my project as I will use an open source Deep Learning transformer model (Frame Semantic Transformer), to investigate its potential to revolutionise how we study history. These types of methods are only just starting to bloom in history and the humanities as a whole, most interesting of which are the developments in semantic and conceptual parsing of historical texts to gain a far broader sense of these trends across time. Most of these attempts have taken hundreds of thousands of texts and used different distributional and probabilistic models to gain significant metadata regarding semantic trends across time. However my model will take a smaller dataset but return semantic data for every sentence in my chosen corpora of writing’s in political thought. The aim is to aggregate this data and see what insights can be gained. What I may lack in total size of my dataset I can hopefully make up for in semantic detail. Therefore I aim to introduce a new model for those working with computational methods for history and I also aim to report on the past, present and future of this field, and its implications for how we study history as a whole.
Approach and Methodology
The inspiration for this project came from studying cognitive linguistics here at LIS. As my background is in history, I saw an avenue of research open up though the combination of cognitive and computational linguistics with history. This came from insights in theories of cognitive linguistics such as Conceptual Metaphor Theory, Relevance Theory and Frame Semantics which all argue that different types of linguistic phenomena are representations of cognitive phenomena that are trying the map and schematise our perception of the world. Through these insights I wondered what implications this could have when trying to understand the semantics of historical texts. I realized that computational methods based on these linguistic theories could give us a far more rigorous understanding of semantic trends in history.
Therefore I decided that FrameNet, a lexical database modelled on the theory of Frame Semantics, could prove useful for this task as the images show from the section above. The Frame Semantic transformer based on FrameNet data, picks out the semantic frames of a sentence which operates as a conceptual scenario that structures semantics.
The data I will use will be texts from history of political thought during and just after the French Revolution in Britain. This is commonly seen as the beginning of the modern era and two central figures, being Edmund Burke and Thomas Paine are, also seen as key founders for conservatism and liberalism with Burke being the former and Paine the latter. They both responded the the revolution in different ways and argued with each other and so this type of analysis for the semantic trends in these authors and their supposed inheritors could prove insightful for demonstrating the potential of this method
Hopefully then at the end of such an analysis we will be able gain some insights into further areas of research in the history of language, ideas and what effects major events in history can have on linguistic phenomena. Such research will, I believe be of interest to historians and linguistics.
Proposal/Outcome
Beyond Outcomes
Want to learn more about this project?

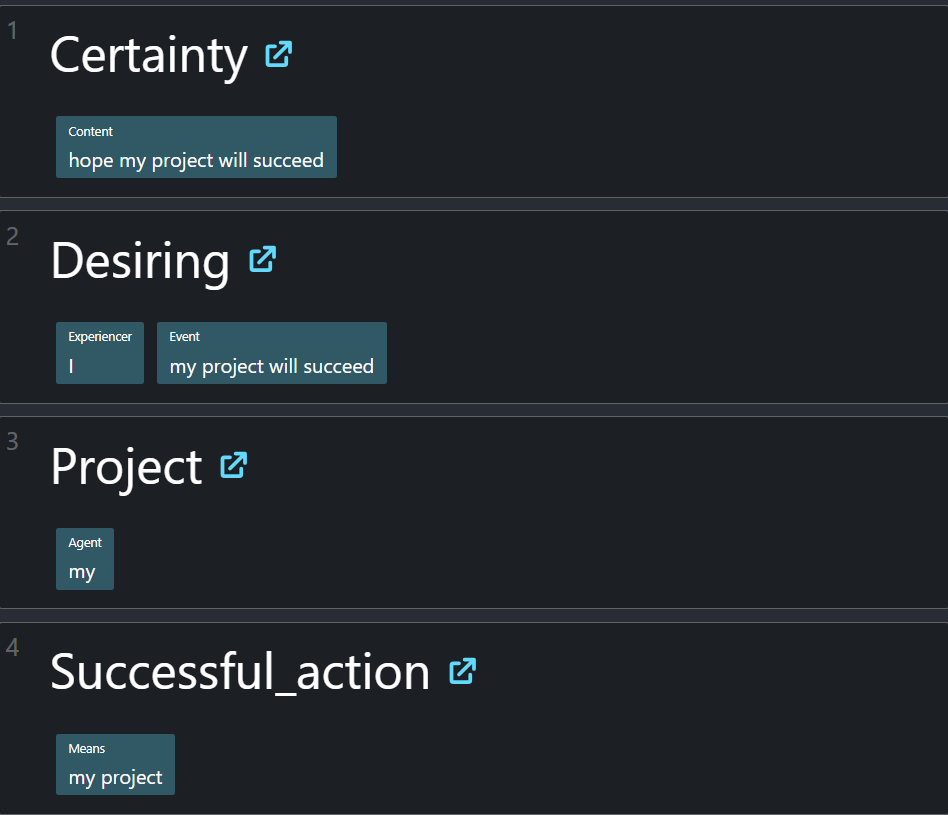
Overall LIS Journey
What are the possibilities of Computational methods, such as NLP and Deep Learning AI, for the study of history? This question is at the heart of my project as I will use an open source Deep learning transformer model (Frame Semantic Transformer), to investigate its potential to revolutionise how we study history. These types of methods are only just starting to bloom in history and the humanities as a whole, most interesting of which are the developments in semantic and conceptual parsing of historical texts to gain a far broader sense of these trends across time. Most of these attempts have taken hundreds of thousands of texts and used different distributional and probabilistic models to gain significant metadata regarding semantic trends across time. However my model will take a smaller dataset but return semantic data for every sentence in my chosen corpora of writing’s in political thought. The aim is to aggregate this data and see what insights can be gained. What I may lack in total size of my dataset I can hopefully make up for in semantic detail. Therefore I aim to introduce a new model for those working with computational methods for history and I also aim to report on the past, present and future of this field.
Other Related Projects
Back to the repository